Swimming lessons
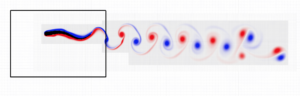
A vorticity model of a swimming eel. (Image: After Nangia et al., Northwestern University and Lawrence Berkeley National Laboratory, from “A moving control volume approach to computing hydrodynamic forces and torques on immersed bodies,” arXiv.1704.00239v2, 15 June 2017.)
Engineers have developed a menagerie of robotic fish for marine biologists, educators, toy makers and even the CIA. But none of these inventions swim as well as their live counterparts, because no one fully understands the physics of how fish propel themselves through water.
Amneet Pal Singh Bhalla netted that slippery subject while he was a postdoctoral scholar at Lawrence Berkeley National Laboratory. He is now assistant professor of mechanical engineering at San Diego State University.
While a mechanical engineering Ph.D. student at Northwestern University, he developed a new approach to simulate how fluid swirls around a fish or any other solid object. With this new approach, Bhalla and his colleagues have resolved some longstanding challenges in understanding how fluids interact with solid structures. Focusing on the knifefish, a creature with a distinctive, body-length fin that undulates like a ribbon, they teased apart the complex interplay between thrust and drag for a swimming fish. They’ve also examined features such as fin height and movement patterns that evolution has optimized for peak swimming efficiency.
But Bhalla’s simulations could be used to study more complex flows in many applications, including renewable-energy devices. Improving how solid objects move through fluids could boost the development of next-generation underwater vehicles, wave-energy conversion devices, windmill blades and more, he says.
Computer simulations can be faster and more efficient than physical experiments to test new designs. But simulating fluid-structure interactions, or FSI, “and computing hydrodynamic forces is challenging, especially with complicated geometries,” Bhalla says.
Previous fish motion simulations involved developing a curved grid fitted to the fish’s body. Researchers then modeled that body’s undulations as it moved through the computational grid. But simulations also had to account for currents and eddies the fish’s motion created in the surrounding water. Simultaneously modeling these complex, interdependent features hogs computing resources and limits the size and scale of systems researchers can test.
Working with Northwestern’s Neelesh Patankar, Bhalla borrowed a well-known technique called immersed boundary and combined it with another strategy known as control volume analyses. Instead of simulating a moving body’s entire surface in fine detail, the researchers place the simulated fish within a flat-sided control volume – a box shape – surrounded by ocean that is divided into cube units in what’s called a Cartesian grid. Researchers can calculate momentum changes within the volume the fish occupies and across the boundaries, and they can use that information to determine the hydrodynamic forces acting on and responding to the fish inside.
Instead of investing in a computationally expensive fish-conforming mesh, the method focuses on the forces and torques the fluid induces on a simplified box that surrounds the swimming fish. It “uses a mathematical trick to compute hydrodynamic forces and torques on complex geometries, which is very efficient yet simple,” Bhalla says. From the actions of the fluid, the team can infer the fluid-structure interactions, such as thrust and drag.
A key innovation in Bhalla’s technique is that the control volume moves along with the fish as it swims, like a video camera tracking a runner. The movement keeps the control volume box oriented to the larger grid, which simplifies calculations that trace currents and eddies flowing out of the box and into the surroundings. Within the control volume, Bhalla uses adaptive mesh refinement (AMR) to produce small and large patches of Cartesian grid with changing resolutions. These patches capture details of the hydrodynamic forces and torques around the fish.
To validate the method, the group identified which parts of a swimming fish generate thrust and which cause drag. “When we see an airplane flying or a boat that cruises on the surface of water, we can easily identify the drag- and thrust-producing regions,” Bhalla says. Propellers and jet engines produce thrust, and fuselages and hulls cause drag. “How about a fish, like an eel, that swims by undulating its body? Which part of its body causes drag and which part produces thrust? This is not a trivial question.”
In fish, the same body parts that cause drag also produce thrust. The team simulated the movements of and forces surrounding the long, continuous ribbon fin along the bottom of the black ghost knifefish’s body. Large wave shapes rippling backward along the fin push on the water, thrusting the fish forward. But by zooming in close to the fin, the simulation showed the fin’s surface also pulls nearby water forward, creating drag.
Building on these insights, the team can also predict the body features and movement patterns that maximize the swimming efficiency of live fish. When the team simulated swimming of various-sized knifefish, it discovered that the most efficient fin height was approximately 1 centimeter. The predictions matched the actual fin heights from 13 diverse knifefish species.
The team also studied 22 species that swim like the knifefish, by rippling fins along the body instead of swishing their tails. The researchers compared the length of fin undulations (wavelength) to the side-to-side motion (amplitude). In all cases, the wavelength was about 20 times larger than the amplitude. This optimal ratio emerged separately in eight distinct vertebrate and invertebrate family trees, revealing a remarkable case of convergent evolution – a problem for which natural selection always seems to give the same solution.
The optimal ratio holds true for swimming robots. Using a robotic fish called Ghostbot, invented by Northwestern’s Malcolm MacIver, the team showed that a robo-fish with the 20:1 ratio swam most efficiently in an experimental flow tunnel. “Therefore, we can design a fish-bot that can generate the maximum amount of thrust by controlling its body deformations in a pre-calculated manner,” Bhalla says.
The finding adds another piece to Bhalla’s long-term goal: “to create numerical methods for FSI in our open-source code that can be used to do complex computational fluid dynamics modeling of industrial processes. The open-source tool should be highly scalable yet flexible enough to be used by the research community with relative ease.”
About the Author
Andy Boyles is a senior science writer at the Krell Institute and contributing science editor at Highlights for Children Inc.