Decoding disease
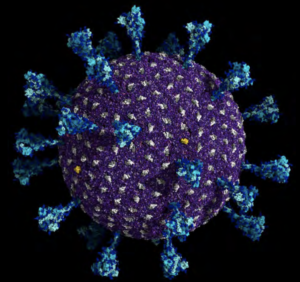
A molecular representation of the delta SARS-CoV-2 all-atom model. Spike proteins are colored in cyan; viral membrane proteins and viral envelope proteins are depicted in gray and yellow, respectively. The viral membrane is in purple. (Image: Lorenzo Casalino and Abigail Dommer, Amaro Lab at the University of California San Diego.)
Anda Trifan, a multilingual Romanian émigré, planned on an international business career and chose DePaul University for its strength in the subject.
But “about a week before school started, I called my counselor and changed all my courses to science courses,” thinking she might want to attend medical school, she says. “I just felt like I wouldn’t be happy doing business.”
The Department of Energy Computational Science Graduate Fellowship (DOE CSGF) recipient completed her degree in chemistry – a subject she hated as a middle schooler in the Chicago suburbs – and fell in love with research, turning her away from medicine. Fortunately, she’s still seeking cures while studying theoretical and computational biophysics at the University of Illinois at Urbana-Champaign (UIUC). She’s happy she switched majors.
Trifan and her advisor, Emad Tajkhorshid, use computation to penetrate cellular machinery. Experiments are indispensable, but they can only produce static high-resolution images of molecules, which are constantly moving, rotating around their bonds to produce different shapes known as conformations. “For example, if you wanted to see the dynamics of how a drug binds to a protein, you’re unable to really do that” via experiments, Trifan says. “The methodology of what we do, for me, is extremely powerful.”
Trifan focuses on Ras, a protein that helps regulate how cells divide, grow and eventually die. Mutated forms can lead to persistently multiplying cells, causing cancers in the pancreas, colon, lungs and other organs. Her DOE CSGF practicum, meanwhile, drew Trifan into battle against another health scourge: COVID-19. Her three-month Argonne National Laboratory stay was extended to an internship, producing essential insights and award-winning research.
Tajkhorshid, the J. Woodland Hastings Endowed Chair in Biochemistry, directs the National Institutes of Health Biomedical Technology Resource for Macromolecular Modeling and Bioinformatics. The center develops and uses two main programs: the nanoscale molecular dynamics (NAMD) code, and VMD, or visual molecular dynamics, which generates images from the simulations.
Understanding Ras mutations “is a very important but a very challenging problem,” Tajkhorshid says. The protein is part of a signaling cascade in cells and functions at the surface of their plasma membranes. Ras works through multiple pathways, so blocking just one may not disable it. Atom-level “studies like molecular dynamics are perfect for studying Ras because you’re able to see how mutations affect behavior” at the membrane’s surface, Trifan says.
The Ras subunit governing cell proliferation tethers to the membrane via a flexible linker. Mutations in the protein can keep cells’ growth and division signals turned on, spurring cells to become cancerous. Trifan simulated the full Ras protein on Anton 2 at the Pittsburgh Supercomputing Center and showed that this linker helps Ras orient itself on the membrane, optimizing interactions with partner proteins in the signaling pathway. Understanding Ras’s functional components could help find cancer treatments.
A mutual interest in Ras prompted Trifan to join Arvind Ramanathan’s Argonne group for her practicum in January 2020. The team was developing machine learning (ML) techniques to more efficiently sift data from simulations of protein-membrane interactions. Trifan modified the group’s method to distribute time- and memory-consuming model training over multiple graphics processing units (GPUs), which speed calculations in high-performance computing systems.
The practicum was nearly over when dire pandemic forecasts forced business and school closures. Ramanathan’s group turned to studying the SARS-CoV-2 virus via simulation, joining with researchers across DOE in a multifaceted consortium. Trifan stayed on, collaborating virtually while pursuing her doctoral research and giving birth to a daughter.
“It was absolutely crazy,” with constant meetings and late nights, Trifan says. “I loved it. It was one of the most exciting times of my career, and I appreciate the opportunity.”
Trifan’s biophysics knowledge complemented the Argonne group’s artificial intelligence expertise, but her tenacity also was an asset, Ramanathan says. “Once you give her a problem, she won’t rest until it’s done.”
Trifan contributed to many COVID-19 research advances. The biggest success was a collaboration with Rommie Amaro’s University of California San Diego (UCSD) biochemistry lab and others to probe the virus’s spike protein complex, the main infection mechanism.
The UCSD team ran a 305-million-atom virus model on Oak Ridge National Laboratory’s Summit, among the world’s most powerful machines. The Argonne group’s AI workflow identified rare spike protein conformation changes in the results, accelerating the model by 10 times. Trifan took “a leading role on the biophysics side, trying to explain how the spike binds to the cell surface,” Ramanathan says. The project won a COVID19-focused version of the 2020 Association for Computing Machinery Gordon Bell Prize for outstanding achievement.
Trifan is even prouder of two 2021 Bell Prize finalist papers the collaboration produced. She was first author on one, a simulation of the virus’s replication-transcription complex (RTC), which copies genetic instructions enabling viral reproduction. Even cutting-edge experimental techniques such as cryogenic electron microscopy (cryo-EM), which averages multiple two-dimensional pictures, fail to capture RTC structural details or evolution. Researchers also discard terabytes of cryo-EM data. All-atom MD simulations, meanwhile, are precise but often too demanding to track the entire RTC.
The team “tried to do something that hasn’t really been done before,” Trifan says: augment the discarded experimental data with multiscale simulations that connect all-atom models’ detail with less-demanding, larger-scale simulations that track RTC interactions over comparatively longer time spans.
The Argonne team ran an all-atom RTC model, with Trifan coordinating efforts and filling data gaps. The simulations provided input to the larger-scale mesh-based method designed by collaborators at University College London. That method created nodes that blanketed the simulated RTC structure, calculating processes at each to represent the entire complex.
P 11 CAP: A molecular representation of the delta SARS-CoV-2 all-atom model. Spike proteins are colored in cyan; viral membrane proteins and viral envelope proteins are depicted in gray and yellow, respectively. The viral membrane is in purple. Credit: Lorenzo Casalino and Abigail Dommer, Amaro Lab at the University of California San Diego.
For instance, the MD simulation computed protein-interaction values, which were then plugged into the larger-scale model. “You have completely different behavior” when the mesh-based model “is informed with actual data from all-atom simulations,” Trifan says.
The team used AI codes to identify unusual protein conformations and optimized NAMD to run on GPUs. It used Balsam, a workflow manager, to coordinate learning between ThetaGPU at the Argonne Leadership Computing Facility and Perlmutter at DOE’s National Energy Research Scientific Computing Center.
“The goal is to give scientists a more complete picture of the protein’s behavior, not just one static image,” Trifan says. With structures the hybrid code predicts, “we can go back to the 2-D cryo-EM images and put more detail into them.”
The project portrayed, perhaps for the first time, an RNA strand unwinding within the RTC – a key step in proofreading transcriptions of the virus’s genetic code for replication. “That’s important when you want to start treating with drug compounds,” Trifan says. “You have to know how the protein behaves in certain conditions.”
Ramanathan’s group collaborated again with UCSD for the second 2021 Bell Prize finalist paper: portraying a virus-laden aerosol, a breath-emitted droplet millionths of a meter in diameter that can float through rooms and go deep into lungs. The simulations tracked interactions between an unprecedented billion atoms and showed how substances in aerosols help SARS-CoV-2 survive.
The Argonne group steered the macroscopic simulations, using an AI code to find rare protein conformations in weighted ensemble simulations, which increased the odds of identifying such outliers. The simulations found the Delta variant’s spike protein opens its shape up dramatically, Trifan says, perhaps explaining its contagious nature.
Trifan is unsure of her post-graduation plans, but Ramanathan is certain she’s bound for an exceptional career. After all, she’s “one of the few people who can say ‘I was involved in three Gordon Bell submissions, all three made it as finalists, and one of them won.’”
Editor’s note: This article appears in the current issue of DEIXIS: The DOE CSGF Annual.
About the Author
The author is a former Krell Institute science writer.