Molecular landscaping
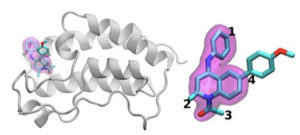
A schematic of the BRD4 protein bound to one of 16 drugs based on the same tetrahydroquinoline scaffold (highlighted in magenta). Regions that are chemically modified between the drugs investigated in this study are labeled 1 to 4. Typically, only a small change is made to the chemical structure from one drug to the next. This conservative approach allows researchers to explore why one drug is effective whereas another is not. (Image: Brookhaven National Laboratory.)
Identifying the optimal drug treatment is like hitting a moving target. To stop disease, small-molecule drugs bind tightly to an important protein, blocking its effects in the body. Even approved drugs don’t usually work in all patients. And over time, infectious agents or cancer cells can mutate, rendering a once-effective drug useless.
A core physical problem underlies all these issues: optimizing the interaction between the drug molecule and its protein target. The variations in drug candidate molecules, the mutation range in proteins and the overall complexity of these physical interactions make this work difficult.
Shantenu Jha of the Department of Energy’s (DOE’s) Brookhaven National Laboratory and Rutgers University leads a team trying to streamline computational methods so that supercomputers can take on some of this immense workload. They’ve found a new strategy to tackle one part: differentiating how drug candidates interact and bind with a targeted protein.
For their work, Jha and his colleagues won last year’s IEEE International Scalable Computing Challenge (SCALE) award, which recognizes scalable computing solutions to real-world science and engineering problems.
To design a new drug, a pharmaceutical company might start with a library of millions of candidate molecules that they narrow to the thousands that show some initial binding to a target protein. Refining these options to a useful drug that can be tested in humans can involve extensive experiments to add or subtract atom groups at key locations on the molecule and test how each of these changes alters how the small molecule and protein interact.
Simulations can help with this process. Larger, faster supercomputers and increasingly sophisticated algorithms can incorporate realistic physics and calculate the binding energies between various small molecules and proteins. Such methods can consume significant computational resources, however, to attain the needed accuracy. Industry-useful simulations also must provide quick answers. Because of the tug-of-war between accuracy and speed, researchers are constantly innovating, developing more efficient algorithms and improving performance, Jha says.
This problem also requires managing computational resources differently than for many other large-scale problems. Instead of designing a single simulation that scales to use an entire supercomputer, researchers simultaneously run many smaller models that shape each other and the trajectory of future calculations, a strategy known as ensemble-based computing, or complex workflows.
“Think of this as trying to explore a very large open landscape to try to find where you might be able to get the best drug candidate,” Jha says. In the past, researchers have asked computers to navigate this landscape by making random statistical choices. At a decision point, half of the calculations might follow one path, the other half another.
Jha and his team seek ways to help these simulations learn from the landscape instead. Ingesting and then sharing real-time data is not easy, Jha says, “and that’s what required some of the technological innovation to do at scale.” He and his Rutgers-based team are collaborating with Peter Coveney’s group at University College London on this work.
To test this idea, they’ve used algorithms that predict binding affinity and have introduced streamlined versions in a HTBAC framework, for high throughput binding affinity calculator. One such calculator, known as ESMACS, helps them eliminate molecules that bind poorly to a target protein. The other, TIES, is more accurate but more limited in scope and requires 2.5 times more computational resources. Nonetheless, it can help the researchers optimize a promising interaction between a drug and a protein. The HTBAC framework helps them implement these algorithms efficiently, saving the more intensive algorithm for situations where it’s needed.
The team demonstrated the idea by examining 16 drug candidates from a molecule library at GlaxoSmithKline (GSK) with their target, BRD4-BD1 – a protein that’s important in breast cancer and inflammatory diseases. The drug candidates had the same core structure but differed at four distinct areas around the molecule’s edges.
In this initial study the team ran thousands of processes simultaneously on 32,000 cores on Blue Waters, a National Science Foundation (NSF) supercomputer at the University of Illinois at Urbana-Champaign. They’ve run similar calculations on Titan, the Cray XK7 supercomputer at the Oak Ridge Leadership Computing Facility, a DOE Office of Science user facility. The team successfully distinguished between the binding of these 16 drug candidates, the largest such simulation to date. “We didn’t just reach an unprecedented scale,” Jha says. “Our approach shows the ability to differentiate.”
They won their SCALE award for this initial proof of concept. The challenge now, Jha says, is making sure that it doesn’t just work for BRD4 but also for other combinations of drug molecules and protein targets.
If the researchers can continue to expand their approach, such techniques could eventually help speed drug discovery and enable personalized medicine. But to examine more realistic problems, they’ll need more computational power. “We’re in the middle of this tension between a very large chemical space that we, in principle, need to explore, and, unfortunately limited computer resources.” Jha says.
Even as supercomputing expands toward the exascale, computational scientists can more than fill the gap by adding more realistic physics to their models. For the foreseeable future, researchers will need to be resourceful to scale up these calculations. Necessity is the mother of innovation, Jha says, precisely because molecular science will not have the ideal amount of computational resources to carry out simulations.
But exascale computing can help move them closer to their goals. Besides working with University College London and GSK, Jha and his colleagues are collaborating with Rick Stevens of Argonne National Laboratory and the CANcer Distributed Learning Environment (CANDLE) team. This co-design project within the DOE’s Exascale Computing Project is building deep neural networks and general machine-learning techniques to study cancer. The algorithms and software within HTBAC could complement CANDLE’s focus on those approaches.
This broader collaboration among Jha’s group, the CANDLE team and John Chodera’s Lab at Memorial Sloan-Kettering Cancer Center has led to the Integrated and Scalable Prediction of Resistance (INSPIRE) project. This team has already run simulations on DOE’s Summit supercomputer at Oak Ridge National Laboratory. It will soon continue this work on Frontera – the NSF’s leadership machine at the University of Texas at Austin’s Texas Advanced Computing Center.
“We’re hungry for greater progress and greater methodological enhancements,” Jha says. “We’d like to see how these pretty complementary approaches might integratively work toward this grand vision.”
About the Author
Sarah Webb is science media editor at the Krell Institute. She’s managing editor of DEIXIS: The DOE CSGF Annual and producer-host of the podcast Science in Parallel. She holds a Ph.D. in chemistry, a bachelor’s degree in German and completed a Fulbright fellowship doing organic chemistry research in Germany.