Simulation looped in
Department of Energy national laboratories rely on high-performance computing (HPC) simulations and experiments that produce data sets of unprecedented size and complexity. Researchers compare simulation output with experimental data to improve predictions and plan experiments. However, the scale of both experimental and simulated data has moved beyond what humans can handle alone.
Enter CogSim, or cognitive simulation, a new approach for comparing large-scale simulations and experimental data by exploiting new machine-learning (ML) methods in real time, all to design precision experiments that ultimately generate better predictive computations and vice versa.
“The basic idea is to perform compute-driven experimentation and experiment-driven compute” with little distance between the two and real-time communication thanks to “compute that’s co-located with the experiment,” says Lawrence Livermore National Laboratory (LLNL) physicist Brian Spears, principal investigator of the CogSim Initiative and director of the LLNL AI3, a lab-industry-university partnership to develop artificial intelligence applications.
CogSim uses deep neural networks, ML algorithms modeled on brain-cell layouts, to map inputs to outputs, revealing structure in large data sets. The key is to exploit spaces within the data that encode shared characteristics about objects in the world, enabling researchers to evaluate important – and even hidden – features of compressed data.
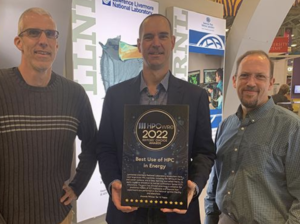
HPCWire’s Editor’s Choice award for best use in energy went to Lawrence Livermore National Laboratory for CogSim. Left to right: LLNL computer scientists Peer-Timo Bremer, Brian Spears and Brian Van Essen accepted the award at the SC22 supercomputing conference last month in Dallas. (Credit: Jeremey Thomas/LLNL.)
CogSim researchers have several strategies to integrate ML into simulation: what they call “in-the-loop” algorithm switching, in which approximations replace complex physics calculations to accelerate simulations; “on-the-loop” prediction and correction that ensures in-flight simulations stay within reasonable bounds; “around-the-loop” learning that proposes the next simulation needed to achieve an optimal data set; and “outside-the-loop” improvements that use ML to produce a simulation-experiment hybrid.
Spears and a handful of other LLNL researchers, including computer scientist Brian Van Essen, began considering machine learning to accelerate the simulation-experiment-analysis loop a few years ago. Van Essen says he and his colleagues “realized that we were co-inventing a thing that was really the same idea, and we were just applying it in stovepipes. Joining our physics and computational teams has made us really powerful.”
CogSim has since risen to the level of an LLNL director’s initiative, with broad financing for a variety of missions. The project’s interdisciplinary team unites experts in ML architectures, deep learning, data harvesting, workflow tools, intelligent data sampling, laser-driven fusion and more.
“Our senior management team has decided that CogSim is a way forward for our national laboratory and probably for the DOE as a whole,” Spears says. “We see CogSim as more than a new software tool. The goal is nothing less than changing how we perform experiments at the national labs.”
Experiments already benefitting from CogSim are internal confinement fusion (ICF) implosions performed at LLNL’s National Ignition Facility (NIF), which uses the world’s largest and most energetic laser. These experiments are costly and time consuming. To boost efficiency, LLNL simulates shots via a multiphysics software package called HYDRA on the Sierra supercomputer. Real-world NIF data are used to validate and fine-tune HYDRA models. This lets the models predict outcomes of real-world experiments more accurately in less time, which can in turn guide the design of live tests. Those ICF advances recently landed LLNL and CogSim an HPCWire Editor’s Choice award for the best use of HPC in energy.
Having learned from NIF’s precise world, the CogSim team has extended its work to high repetition-rate lasers (HRRL) – small but powerful beams that can fire many times per second. “With a laser that operates that quickly, you can’t fire the laser and then think about what you just saw for a hundred milliseconds and then go back and do the next experiment,” Spears says. “So, we built AI sentinels that help us perform the best possible experiments.”
Spears and his colleagues found that ICF and HRRL simulations that would typically take a half-hour to run via standard HPC could be done as well in seconds using CogSim. CogSim technology also has helped in lab-wide research to simulate the spread of SARS-CoV-2, the virus that causes COVID-19. That team effort, supported by military and other federal policymakers, recently won an award from the Weapons and Complex Integration Directorate, recognizing it as an outstanding contribution and achievement supporting the lab’s national security missions.
More recently, the Department of Defense sought help from the team’s bioassurance arm and its lead, Jim Brase, to design new antibodies that protect against the SARS-CoV-2 Omicron variant. Spears says the DoD “came to LLNL and said, ‘We need new antibodies in two weeks and you’re the only shop on the planet that can do this. How fast can you go?’” Spears said. “The answer turned out to be two weeks.” The resulting antibodies are in trials with AstraZeneca.
The COVID-19 work and the ICF tests fit into the first two phases, respectively, of the lab’s focus on discovery, design, manufacturing and deployment. In the manufacturing space, the team is using CogSim to control advanced manufacturing and 3D printers to ensure defects aren’t embedded in parts. In the deploy space, the researchers are building data-driven models of how materials age and whether they’re compatible. “So, if you get design, manufacturing, and deployment right, you don’t make a device that fails a few months after you’ve made it,” Van Essen says.
The initial computing hardware to power CogSim has come from a variety of vendors from established companies like IBM, NVIDIA, HPE and AMD to Cerebras, SambaNova and other emerging players. The lab, working with the companies through its AI3 hub, has integrated application-specific AI accelerators into its HPC systems – the 23-petaflop Lassen and the 11-petaflop Corona clusters – to run AI workloads that the lab has never executed before.
“We are redesigning our HPC codes to offload machine-learning calculations. While the ML work is done on purpose-built AI accelerators, the HPC calculations will continue on GPU machines,” Van Essen says, referring to machines that incorporate graphics chips descended from video games.
Outside of LLNL, CogSim is being applied to a variety of projects at Sandia and Los Alamos national laboratories, including efforts in materials science, uncertainty quantification, weapons physics design and magnetically driven ICF.
Spears sees a big future for CogSim, predicting it “will usher in an era of rapid discovery and science exploration that we couldn’t have thought about with a straight face a decade ago. There’s not much stopping us but the will to go do it.”
About the Author
Chris Palmer has written for more than two dozen publications, including Nature, The Scientist and Cancer Today and for the National Institutes of Health. He holds a doctorate in neuroscience from the University of Texas, Austin, and completed the Science Communication Program at the University of California, Santa Cruz.